Book Downloads Hub Reads Ebooks Online eBook Librarys Digital Books Store Download Book Pdfs Bookworm Downloads Free Books Downloads Epub Book Collection Pdf Book Vault Read and Download Books Open Source Book Library Best Book Downloads Paula Fox Ricardo Aroca Noo Saro Wiwa Margaret Gurevich Daniel M Wegner Barbara Raue Ronald G Musto Vasile Marinca
Do you want to contribute by writing guest posts on this blog?
Please contact us and send us a resume of previous articles that you have written.
Unlocking the Power of Recommender Systems: An Introduction by Alexander Felfernig

In this digital age, where information is abundant and choices are plentiful, it can be overwhelming to sift through the vast sea of options. Whether it's finding the ideal movie to watch, the perfect product to buy, or the right book to read, we often seek guidance to make informed decisions. This is where recommender systems come into play, providing personalized suggestions tailored to our preferences and needs. In this article, we will delve into the fascinating world of recommender systems, exploring the concepts, techniques, and applications, as introduced by renowned expert Alexander Felfernig.
What are Recommender Systems?
Recommender systems are powerful software tools that analyze user behavior and preferences to provide personalized recommendations. By leveraging complex algorithms, these systems can predict user preferences and make intelligent suggestions based on past data. They aim to enhance user experience by reducing information overload and increasing user satisfaction by offering personalized recommendations that suit individual tastes and preferences.
The Role of Alexander Felfernig
Alexander Felfernig is a leading authority in the field of recommender systems, known for his extensive research and contributions in the domain. With decades of experience and numerous research publications, Felfernig has played a pivotal role in advancing the theory and practical implementation of recommender systems.
4.2 out of 5
Language | : | English |
File size | : | 8121 KB |
Text-to-Speech | : | Enabled |
Screen Reader | : | Supported |
Enhanced typesetting | : | Enabled |
Print length | : | 353 pages |
Mass Market Paperback | : | 432 pages |
Lexile measure | : | 1210L |
Item Weight | : | 1.19 pounds |
Dimensions | : | 6.14 x 0.63 x 9.21 inches |
Hardcover | : | 258 pages |

Types of Recommender Systems
There are several types of recommender systems, each relying on different techniques to generate recommendations. The most common ones are:
- Collaborative Filtering: This approach recommends items based on the collective preferences of similar users. It analyzes user behavior and identifies patterns to generate individualized recommendations.
- Content-Based Filtering: This method recommends items based on the characteristics and attributes of the items themselves. It analyses the user's past interactions and recommends items with similar features or content.
- Hybrid Filtering: As the name suggests, this approach combines collaborative filtering and content-based filtering techniques to generate recommendations. It aims to mitigate the limitations of each method individually.
Applications of Recommender Systems
The applications of recommender systems are diverse and widespread, permeating many aspects of our daily lives. Some notable applications include:
- E-commerce: Recommender systems help online retailers suggest personalized products to customers, leading to higher customer satisfaction and increased sales.
- Streaming Services: Platforms like Netflix and Spotify rely heavily on recommender systems to recommend movies, shows, and music to their users, ensuring an enjoyable and tailored experience.
- News and Content Platforms: Recommender systems are crucial in suggesting relevant articles, blog posts, and videos to users on platforms like YouTube and Medium, keeping them engaged and informed.
- Social Media: Social media platforms like Facebook and Instagram leverage recommender systems to recommend friends, groups, and interests based on user behavior and preferences.
- Travel and Accommodation: Recommender systems assist travelers in finding suitable flights, hotels, and destinations based on their preferences and budget.
The Future of Recommender Systems
As technology continues to advance and data becomes more abundant, the future of recommender systems looks promising. The emergence of cutting-edge techniques like deep learning and natural language processing is leading to even more accurate and personalized recommendations. Additionally, the ethical implications of recommender systems are gaining attention, with efforts being made to address issues such as filter bubbles and algorithmic biases.
Recommender systems have revolutionized the way we discover and consume content, products, and services. Alexander Felfernig, with his extensive knowledge and expertise, has significantly contributed to the development and understanding of these systems. As we navigate the vast universe of choices, recommender systems continue to play a vital role in simplifying decision-making processes and enhancing user experiences.
From e-commerce to entertainment and beyond, recommender systems have reshaped industries and empowered both consumers and businesses. By leveraging the power of personalized recommendations, we can unlock a world of possibilities, making our lives easier, more enjoyable, and more efficient.
4.2 out of 5
Language | : | English |
File size | : | 8121 KB |
Text-to-Speech | : | Enabled |
Screen Reader | : | Supported |
Enhanced typesetting | : | Enabled |
Print length | : | 353 pages |
Mass Market Paperback | : | 432 pages |
Lexile measure | : | 1210L |
Item Weight | : | 1.19 pounds |
Dimensions | : | 6.14 x 0.63 x 9.21 inches |
Hardcover | : | 258 pages |
In this age of information overload, people use a variety of strategies to make choices about what to buy, how to spend their leisure time, and even whom to date. Recommender systems automate some of these strategies with the goal of providing affordable, personal, and high-quality recommendations. This book offers an overview of approaches to developing state-of-the-art recommender systems. The authors present current algorithmic approaches for generating personalized buying proposals, such as collaborative and content-based filtering, as well as more interactive and knowledge-based approaches. They also discuss how to measure the effectiveness of recommender systems and illustrate the methods with practical case studies. The final chapters cover emerging topics such as recommender systems in the social web and consumer buying behavior theory. Suitable for computer science researchers and students interested in getting an overview of the field, this book will also be useful for professionals looking for the right technology to build real-world recommender systems.
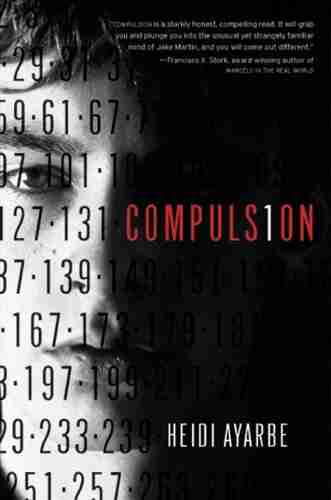

Compulsion Heidi Ayarbe - A Gripping Tale of Addiction...
Compulsion Heidi Ayarbe...
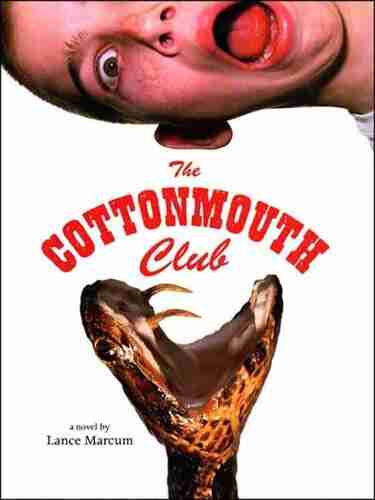

The Cottonmouth Club Novel - Uncovering the Secrets of a...
Welcome to the dark and twisted world of...
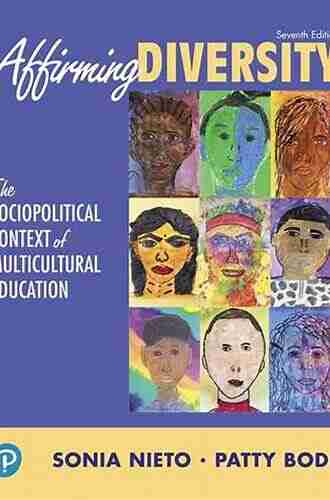

The Sociopolitical Context Of Multicultural Education...
Living in a diverse and interconnected world,...
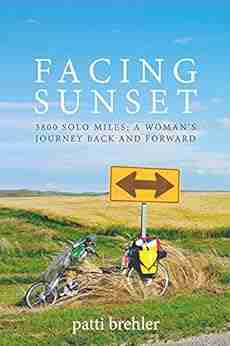

The Epic Journey of a Woman: 3800 Solo Miles Back and...
Embarking on a solo journey is a...
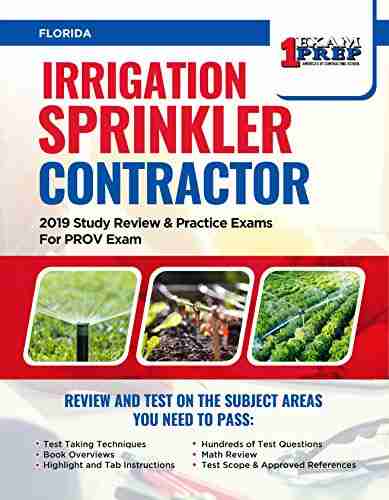

Florida Irrigation Sprinkler Contractor: Revolutionizing...
Florida, known for its beautiful...
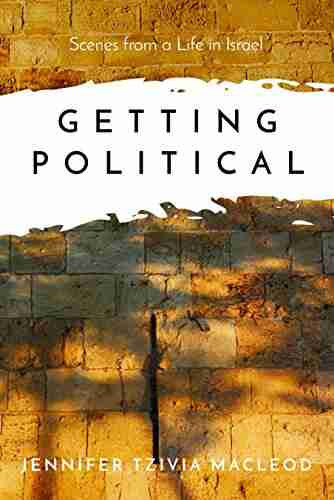

Unveiling the Political Tapestry: Life in Israel
Israel, a vibrant country located in the...
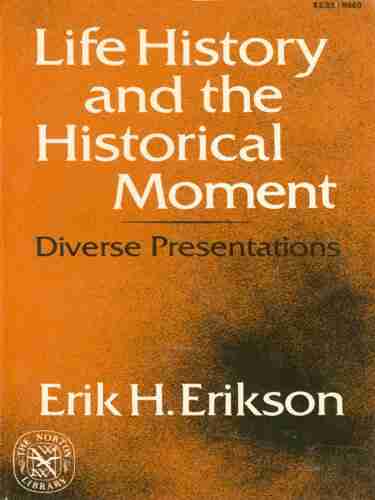

Life History And The Historical Moment Diverse...
Do you ever find yourself...
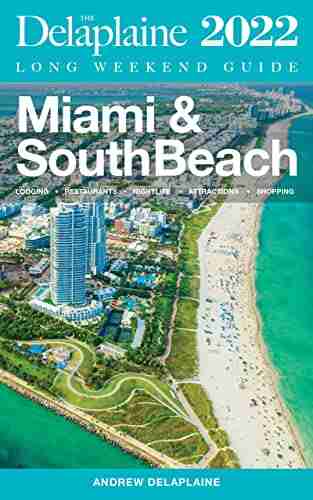

Miami South Beach The Delaplaine 2022 Long Weekend Guide
Welcome to the ultimate guide for...
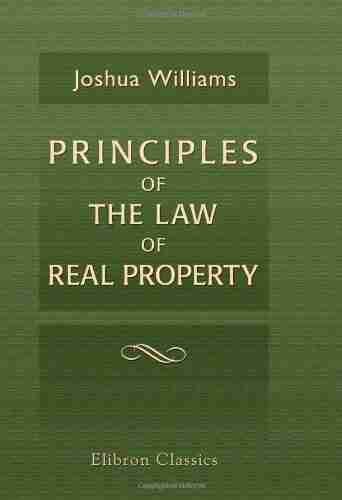

An In-depth Look into the Principles of the Law of Real...
The principles of the...
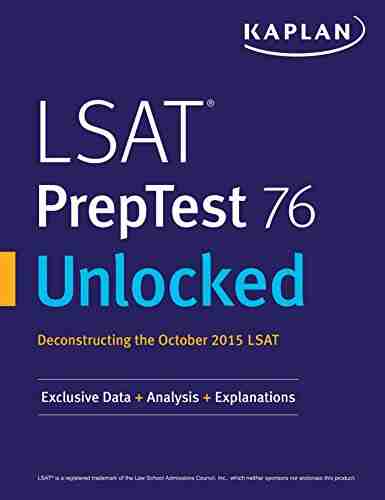

Exclusive Data Analysis Explanations For The October 2015...
Are you preparing for the Law School...
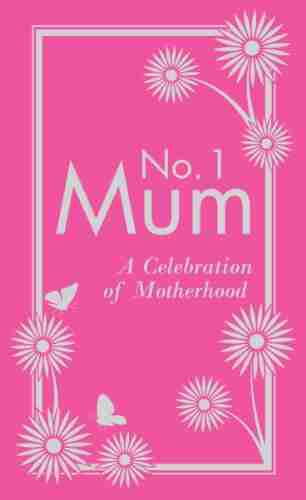

The Secret to Enjoying Motherhood: No Mum Celebration of...
Being a mother is a truly remarkable...
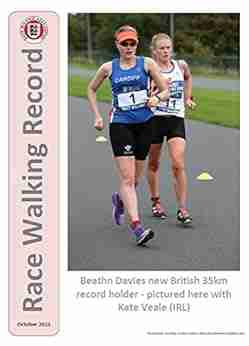

Race Walking Record 913 October 2021
Are you ready for an...
Light bulbAdvertise smarter! Our strategic ad space ensures maximum exposure. Reserve your spot today!
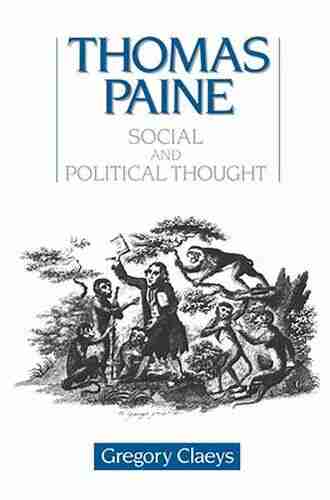

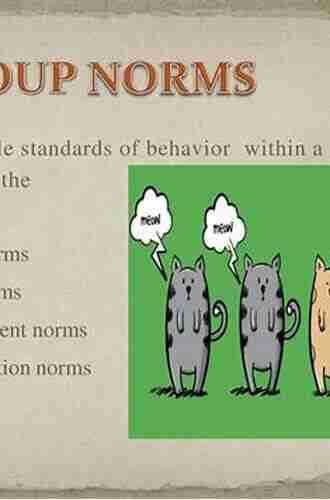

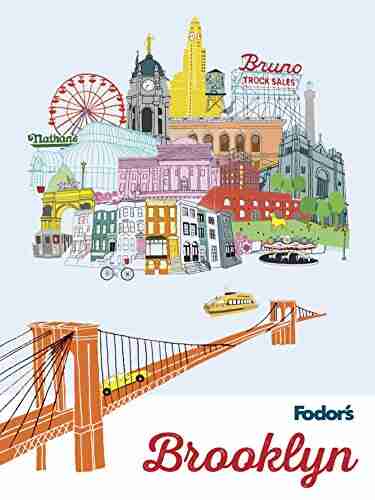

- Lucas ReedFollow ·5.5k
- Quentin PowellFollow ·7k
- Roland HayesFollow ·10.9k
- Gabriel MistralFollow ·7.2k
- Donald WardFollow ·12.5k
- Patrick HayesFollow ·12.8k
- Hassan CoxFollow ·14.3k
- Heath PowellFollow ·16.1k