Book Downloads Hub Reads Ebooks Online eBook Librarys Digital Books Store Download Book Pdfs Bookworm Downloads Free Books Downloads Epub Book Collection Pdf Book Vault Read and Download Books Open Source Book Library Best Book Downloads Bob Deans Alan Kelly Alan Taylor Alejandro Jodorowsky Bruno Luis Carey Martell Patricia Carlin Tess Burrows
Do you want to contribute by writing guest posts on this blog?
Please contact us and send us a resume of previous articles that you have written.
The Natural Language For Artificial Intelligence Cognitive Data Science In

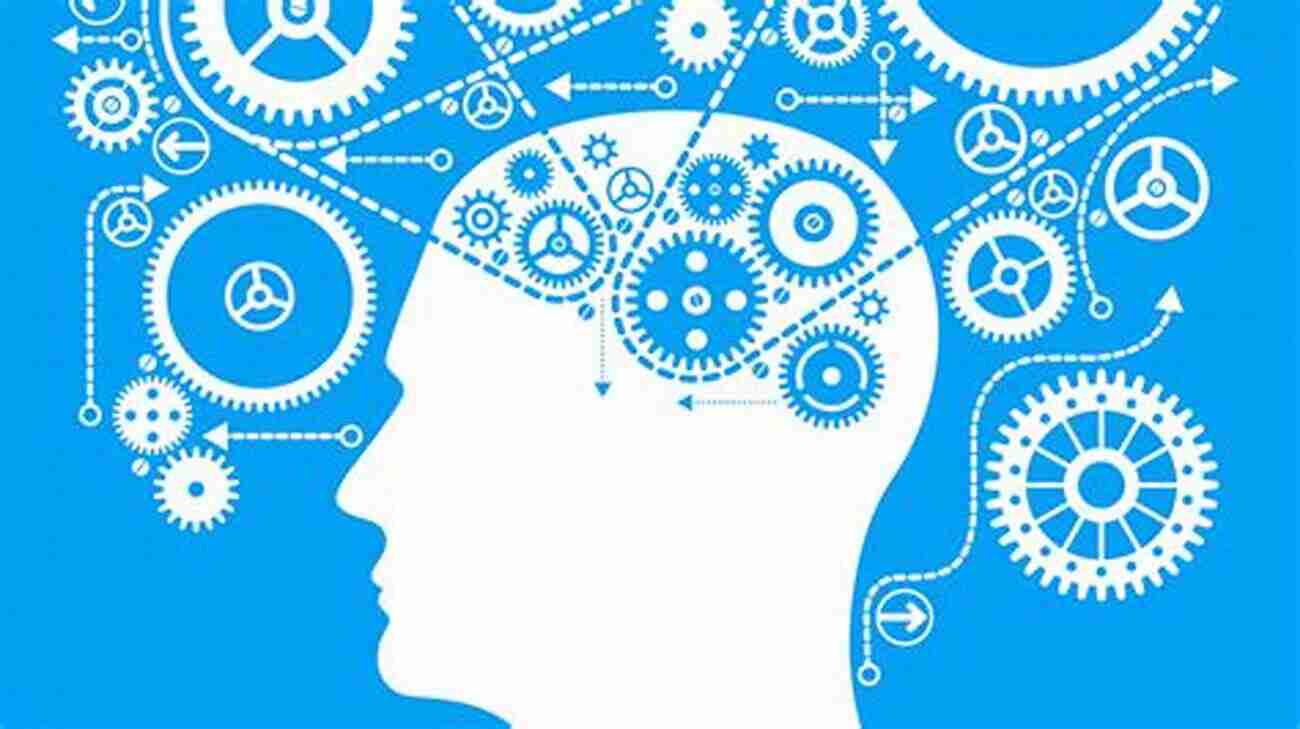
As technology continues to advance, the field of Artificial Intelligence (AI) is making significant strides in various domains. One of the key areas where AI is becoming increasingly prominent is in cognitive data science. In order for AI systems to effectively interpret and analyze vast amounts of data, they need a natural language interface. This article explores the role of natural language in artificial intelligence cognitive data science and its implications for various industries.
Understanding Natural Language Processing
Natural Language Processing (NLP) is a subfield of AI that focuses on enabling machines to understand and process human language. With the help of NLP techniques, AI systems can analyze textual data, extract meaningful insights, and generate responses in a way that resembles human communication. NLP serves as a bridge between humans and machines, enabling cognitive data science to be more interactive and intuitive.
The Importance of Natural Language in Cognitive Data Science
Natural language plays a vital role in cognitive data science for several reasons. Firstly, it facilitates the efficient understanding of complex data sets by allowing users to query data using their natural language. Instead of having to write complex code or SQL queries, users can simply ask questions in plain English. This lowers the barrier to entry for non-technical users and empowers them to extract valuable insights from data without extensive programming knowledge.
4.4 out of 5
Language | : | English |
File size | : | 11714 KB |
Text-to-Speech | : | Enabled |
Screen Reader | : | Supported |
Enhanced typesetting | : | Enabled |
Print length | : | 254 pages |
Paperback | : | 24 pages |
Item Weight | : | 3.68 ounces |
Dimensions | : | 8 x 0.06 x 10 inches |
Secondly, natural language provides a more interactive and engaging experience when dealing with AI systems. By incorporating conversational interfaces, users can have a dialogue with AI-powered cognitive data science platforms, making the process more intuitive and efficient. This fosters collaboration between humans and machines, enabling teams to make informed decisions and discover patterns that might have been overlooked using traditional data science approaches.
Applications of Natural Language in Cognitive Data Science
The incorporation of natural language in cognitive data science has numerous applications across various industries. In healthcare, for example, physicians can use natural language queries to analyze patient records and identify potential risk factors for diseases. This streamlines the diagnosis process and enables healthcare professionals to provide more personalized and accurate treatment plans.
In finance, natural language interfaces can be utilized to analyze market trends, news, and social media sentiments to make informed investment decisions. Traders can ask questions about asset performance, calculate risk metrics, and identify patterns in the market, enabling more effective trading strategies.
Natural language interfaces also have significant applications in customer support. Chatbots equipped with NLP capabilities can understand and respond to customer inquiries, providing personalized assistance and resolving issues in real-time. This enhances customer satisfaction and improves overall service efficiency.
The Future of Natural Language in Cognitive Data Science
With advancements in AI and NLP, the future of natural language in cognitive data science looks promising. More sophisticated AI models are being developed that can comprehend context, tone, and user intent, providing more accurate and human-like responses. Additionally, as the technology becomes more accessible and user-friendly, natural language interfaces will become a standard feature in cognitive data science platforms, democratizing data analysis and fostering innovation across industries.
Natural language is revolutionizing the field of cognitive data science by enabling AI systems to understand, process, and communicate in human language. The incorporation of natural language interfaces empowers users to extract valuable insights from complex data sets and facilitates collaboration between humans and machines. With the applications ranging from healthcare to finance, the future of natural language in cognitive data science is bound to bring further advancements, making data analysis more intuitive and impactful than ever before.
4.4 out of 5
Language | : | English |
File size | : | 11714 KB |
Text-to-Speech | : | Enabled |
Screen Reader | : | Supported |
Enhanced typesetting | : | Enabled |
Print length | : | 254 pages |
Paperback | : | 24 pages |
Item Weight | : | 3.68 ounces |
Dimensions | : | 8 x 0.06 x 10 inches |
The Natural Language for Artificial Intelligence presents the biological and logical structure typical of human language in its dynamic mediating process between reality and the human mind. The book explains linguistic functioning in the dynamic process of human cognition when forming meaning. After that, an approach to artificial intelligence (AI) is outlined, which works with a more restricted concept of natural language that leads to flaws and ambiguities. Subsequently, the characteristics of natural language and patterns of how it behaves in different branches of science are revealed to indicate ways to improve the development of AI in specific fields of science.
A brief description of the universal structure of language is also presented as an algorithmic model to be followed in the development of AI. Since AI aims to imitate the process of the human mind, the book shows how the cross-fertilization between natural language and AI should be done using the logical-axiomatic structure of natural language adjusted to the logical-mathematical processes of the machine.
- Presents a comprehensive approach to natural language and its inherent and complex dynamics
- Develops language content as the next frontier, identifying the universal structure of language as a common structure that appears in both AI and cognitive computing
- Explains the standard structure present in cognition and AI, making them interchangeable
- Offers examples of the application of the universal language model in image analysis and conventional language
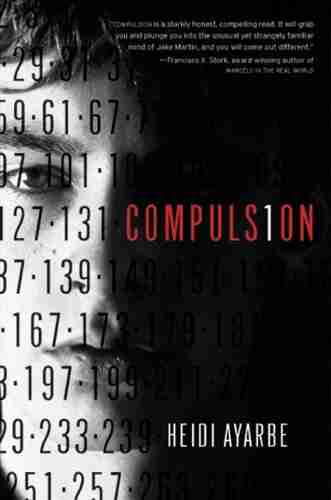

Compulsion Heidi Ayarbe - A Gripping Tale of Addiction...
Compulsion Heidi Ayarbe...
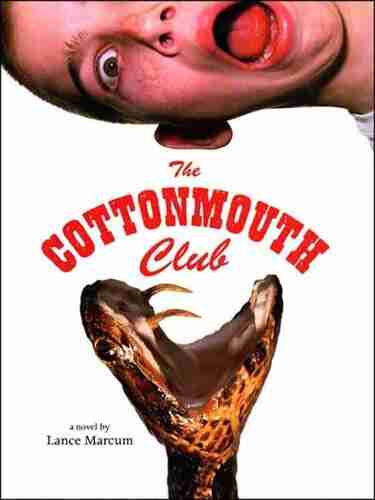

The Cottonmouth Club Novel - Uncovering the Secrets of a...
Welcome to the dark and twisted world of...
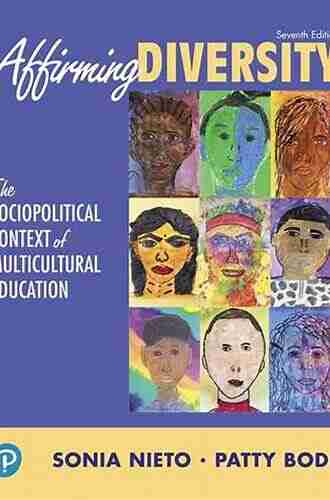

The Sociopolitical Context Of Multicultural Education...
Living in a diverse and interconnected world,...
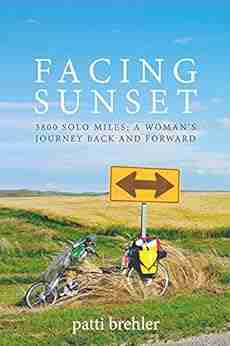

The Epic Journey of a Woman: 3800 Solo Miles Back and...
Embarking on a solo journey is a...
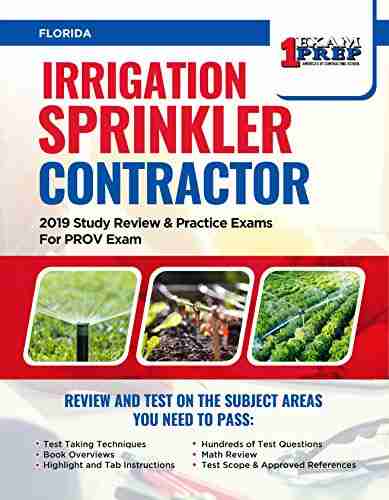

Florida Irrigation Sprinkler Contractor: Revolutionizing...
Florida, known for its beautiful...
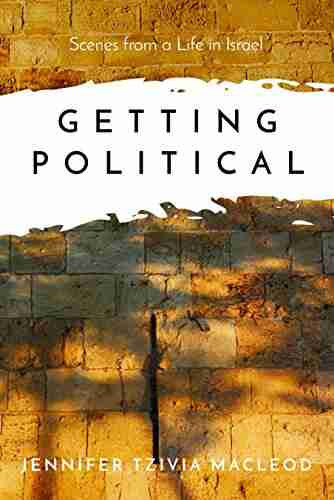

Unveiling the Political Tapestry: Life in Israel
Israel, a vibrant country located in the...
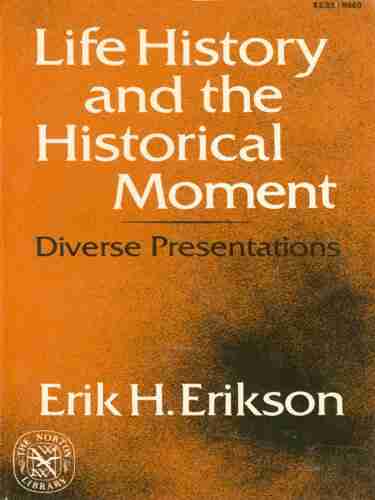

Life History And The Historical Moment Diverse...
Do you ever find yourself...
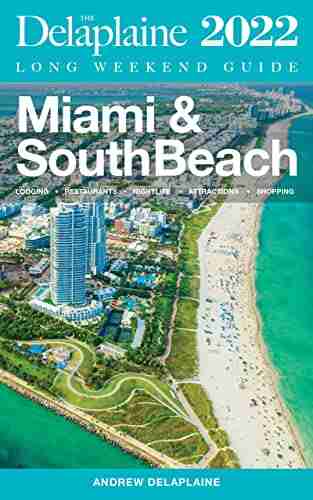

Miami South Beach The Delaplaine 2022 Long Weekend Guide
Welcome to the ultimate guide for...
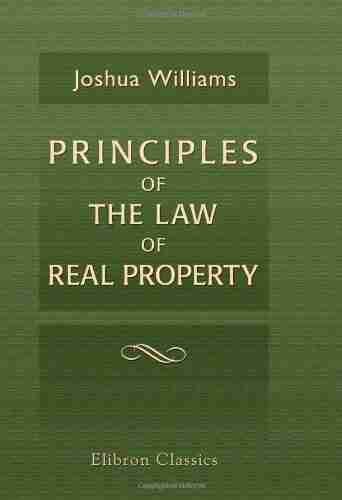

An In-depth Look into the Principles of the Law of Real...
The principles of the...
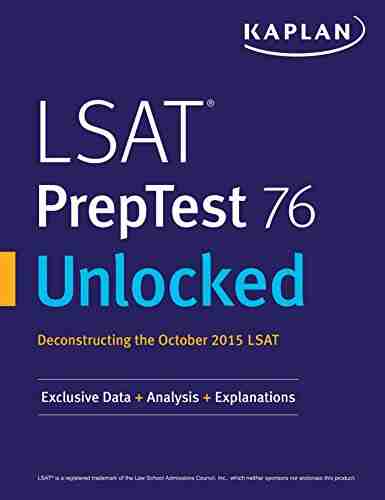

Exclusive Data Analysis Explanations For The October 2015...
Are you preparing for the Law School...
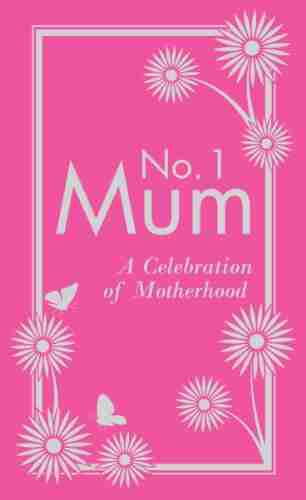

The Secret to Enjoying Motherhood: No Mum Celebration of...
Being a mother is a truly remarkable...
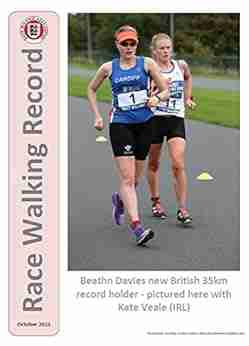

Race Walking Record 913 October 2021
Are you ready for an...
Light bulbAdvertise smarter! Our strategic ad space ensures maximum exposure. Reserve your spot today!
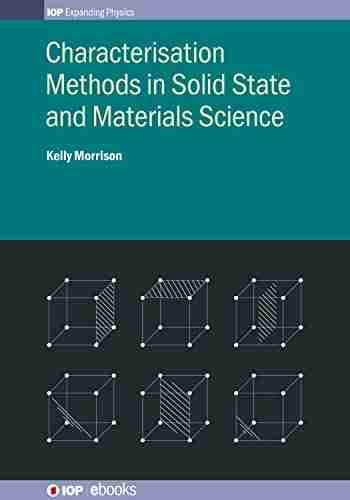

- Isaias BlairFollow ·5.8k
- Chandler WardFollow ·2.2k
- Ross NelsonFollow ·9.7k
- Tyler NelsonFollow ·15.1k
- Edward ReedFollow ·14k
- Jayson PowellFollow ·16.9k
- Robbie CarterFollow ·15.6k
- Ricky BellFollow ·13.1k