Book Downloads Hub Reads Ebooks Online eBook Librarys Digital Books Store Download Book Pdfs Bookworm Downloads Free Books Downloads Epub Book Collection Pdf Book Vault Read and Download Books Open Source Book Library Best Book Downloads Kathy Long Georges St Pierre Herbert Gintis Maureen Cashman Julian Baggini Alberto Clemente De La Torre William Madden Iacob Adrian
Do you want to contribute by writing guest posts on this blog?
Please contact us and send us a resume of previous articles that you have written.
Reinforcement Learning For Optimal Feedback Control: Unlocking New Frontiers in Intelligent Systems

Are you ready to dive deep into the world of reinforcement learning and its application in optimizing feedback control systems? In this article, we will explore the exciting field of reinforcement learning for optimal feedback control and how it is revolutionizing the way intelligent systems operate.
Understanding Feedback Control Systems
Before we delve into the intricacies of reinforcement learning, let's first gain a clear understanding of feedback control systems. In simple terms, a feedback control system involves continuously monitoring the output of a process and comparing it to the desired or reference output. The system then adjusts its inputs based on this feedback to maintain or achieve the desired performance.
Feedback control systems have been extensively used in various fields, including manufacturing, robotics, automatic pilot systems, and even biological systems. The ability to automatically adapt and optimize the control inputs based on feedback is crucial for achieving desired outcomes and maintaining stability.
4 out of 5
Language | : | English |
File size | : | 79751 KB |
Text-to-Speech | : | Enabled |
Enhanced typesetting | : | Enabled |
Word Wise | : | Enabled |
Print length | : | 586 pages |
Screen Reader | : | Supported |
Hardcover | : | 422 pages |
Item Weight | : | 5.1 ounces |
Dimensions | : | 5.5 x 0.17 x 8.5 inches |
Paperback | : | 72 pages |
The Role of Reinforcement Learning
In recent years, reinforcement learning (RL) has emerged as a powerful technique for optimizing feedback control systems. RL is a subfield of machine learning that focuses on how an agent can learn to interact with an environment to maximize cumulative rewards.
Traditionally, feedback control systems relied on predefined control algorithms designed by human experts. While these algorithms worked well for many applications, they often struggled in complex and dynamic environments where expert knowledge may be limited or insufficient.
RL, on the other hand, allows agents to learn optimal control policies directly from experience. By interacting with the environment and receiving feedback in the form of rewards or penalties, the agent can update its policy to maximize long-term rewards. This ability to learn and adapt over time enables RL-based feedback control systems to perform exceptionally well in complex and uncertain environments.
Applications of Reinforcement Learning in Feedback Control
The applications of reinforcement learning in feedback control are vast and diverse. Let's explore a few examples to highlight the potential impact of RL in various domains.
Robotic Control
Robots operating in unstructured environments face numerous challenges, such as object manipulation, navigation, and grasping. RL can enable robots to learn optimal control policies through trial and error, leading to enhanced performance and adaptability. For instance, RL has been used to teach robots how to walk, fly, and perform complex tasks autonomously.
Power Grid Management
The electricity grid is a complex and dynamic system that requires efficient control to ensure stability and minimize disruptions. Reinforcement learning algorithms can optimize control actions such as generation schedules, voltage regulation, and load balancing to improve grid stability and reduce operational costs.
Automated Trading
In the world of high-frequency trading, RL algorithms are widely used to make quick and accurate predictions based on market data and optimize trading strategies. These algorithms continuously adapt to changing market conditions, helping traders maximize profits and reduce risks.
Challenges and Future Directions
Despite the numerous successes of reinforcement learning in feedback control, there are still several challenges that need to be addressed. One such challenge is the sample complexity of RL algorithms, as they often require a large number of interactions with the environment to learn optimal control policies. Additionally, ensuring safety and stability in RL-based control systems is another critical area that needs further research.
Looking ahead, the future of reinforcement learning in feedback control seems promising. Researchers are actively exploring novel RL algorithms, combining them with other optimization techniques, and integrating them into existing control frameworks to further enhance the performance and applicability of intelligent systems.
Reinforcement learning for optimal feedback control is transforming the way intelligent systems operate across various domains. By allowing agents to learn optimal control policies through interaction with the environment, RL enables intelligent systems to adapt and thrive in complex and uncertain environments.
As we continue to unlock new frontiers in reinforcement learning, the potential for even greater advancements in intelligent systems becomes more apparent. With ongoing research and development, we can expect RL-based feedback control systems to revolutionize industries and pave the way for a more intelligent future.
4 out of 5
Language | : | English |
File size | : | 79751 KB |
Text-to-Speech | : | Enabled |
Enhanced typesetting | : | Enabled |
Word Wise | : | Enabled |
Print length | : | 586 pages |
Screen Reader | : | Supported |
Hardcover | : | 422 pages |
Item Weight | : | 5.1 ounces |
Dimensions | : | 5.5 x 0.17 x 8.5 inches |
Paperback | : | 72 pages |
Reinforcement Learning for Optimal Feedback Control develops model-based and data-driven reinforcement learning methods for solving optimal control problems in nonlinear deterministic dynamical systems. In order to achieve learning under uncertainty, data-driven methods for identifying system models in real-time are also developed. The book illustrates the advantages gained from the use of a model and the use of previous experience in the form of recorded data through simulations and experiments. The book’s focus on deterministic systems allows for an in-depth Lyapunov-based analysis of the performance of the methods described during the learning phase and during execution.
To yield an approximate optimal controller, the authors focus on theories and methods that fall under the umbrella of actor–critic methods for machine learning. They concentrate on establishing stability during the learning phase and the execution phase, and adaptive model-based and data-driven reinforcement learning, to assist readers in the learning process, which typically relies on instantaneous input-output measurements.
This monograph provides academic researchers with backgrounds in diverse disciplines from aerospace engineering to computer science, who are interested in optimal reinforcement learning functional analysis and functional approximation theory, with a good to the use of model-based methods. The thorough treatment of an advanced treatment to control will also interest practitioners working in the chemical-process and power-supply industry.
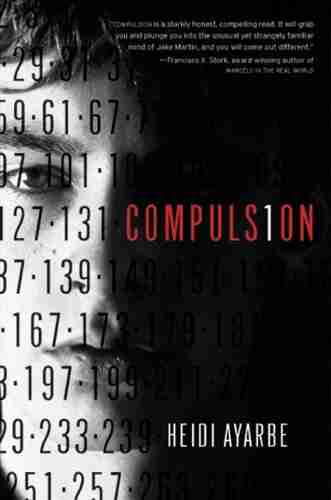

Compulsion Heidi Ayarbe - A Gripping Tale of Addiction...
Compulsion Heidi Ayarbe...
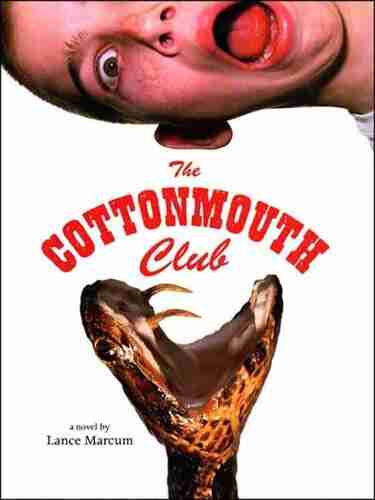

The Cottonmouth Club Novel - Uncovering the Secrets of a...
Welcome to the dark and twisted world of...
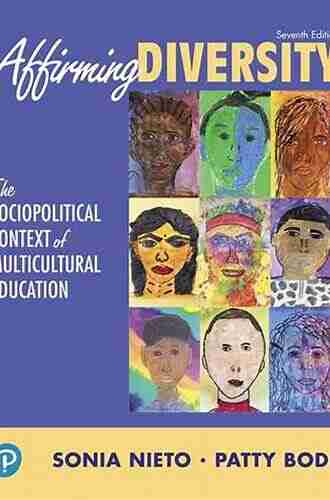

The Sociopolitical Context Of Multicultural Education...
Living in a diverse and interconnected world,...
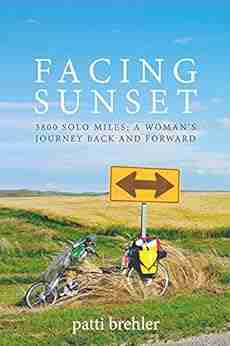

The Epic Journey of a Woman: 3800 Solo Miles Back and...
Embarking on a solo journey is a...
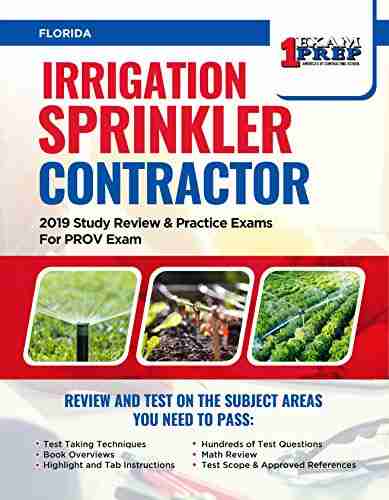

Florida Irrigation Sprinkler Contractor: Revolutionizing...
Florida, known for its beautiful...
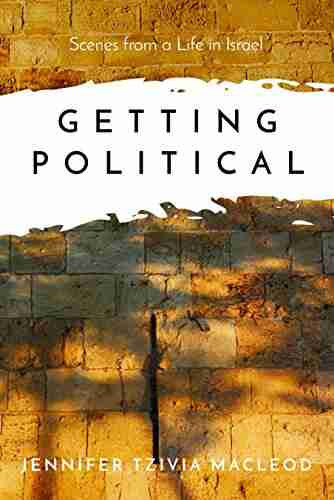

Unveiling the Political Tapestry: Life in Israel
Israel, a vibrant country located in the...
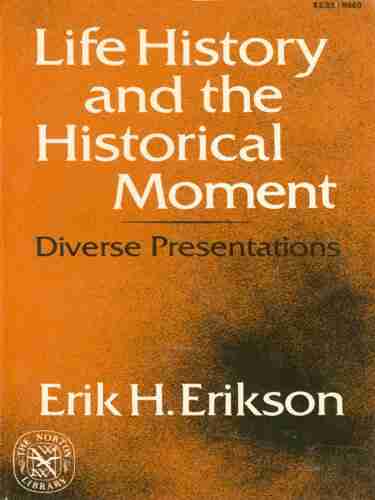

Life History And The Historical Moment Diverse...
Do you ever find yourself...
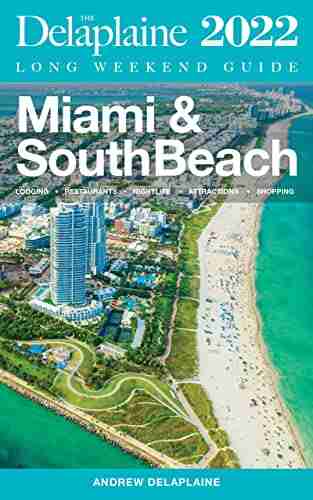

Miami South Beach The Delaplaine 2022 Long Weekend Guide
Welcome to the ultimate guide for...
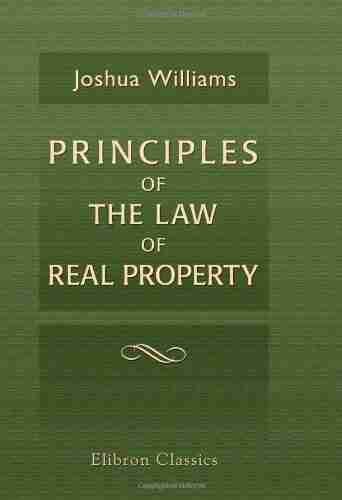

An In-depth Look into the Principles of the Law of Real...
The principles of the...
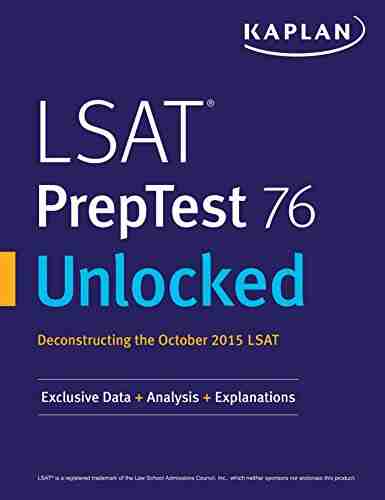

Exclusive Data Analysis Explanations For The October 2015...
Are you preparing for the Law School...
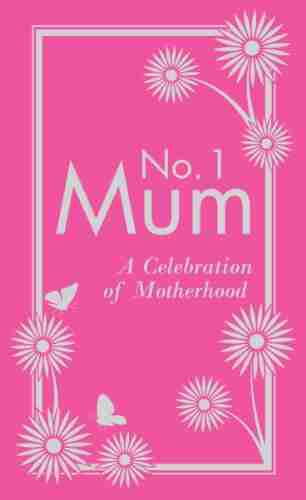

The Secret to Enjoying Motherhood: No Mum Celebration of...
Being a mother is a truly remarkable...
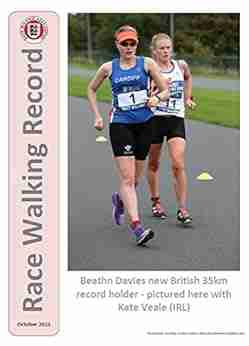

Race Walking Record 913 October 2021
Are you ready for an...
Light bulbAdvertise smarter! Our strategic ad space ensures maximum exposure. Reserve your spot today!
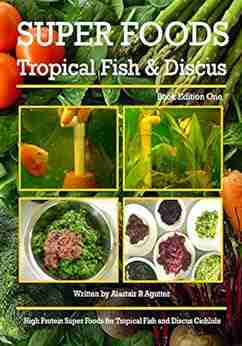

- Jace MitchellFollow ·12.4k
- Roy BellFollow ·4.5k
- Marc FosterFollow ·15.5k
- Vic ParkerFollow ·16.3k
- Henry Wadsworth LongfellowFollow ·17.8k
- Joseph FosterFollow ·4.7k
- Carson BlairFollow ·8.7k
- Herbert CoxFollow ·9.7k