Book Downloads Hub Reads Ebooks Online eBook Librarys Digital Books Store Download Book Pdfs Bookworm Downloads Free Books Downloads Epub Book Collection Pdf Book Vault Read and Download Books Open Source Book Library Best Book Downloads Cynthia A Rodriguez Kevin Timpe J A Tyler Angela M Smith Richard Slotkin Simon Grabowsky Paul G Walmsley Chris Seiter
Do you want to contribute by writing guest posts on this blog?
Please contact us and send us a resume of previous articles that you have written.
Optical Flow And Trajectory Estimation Methods - A Phenomenal Breakthrough

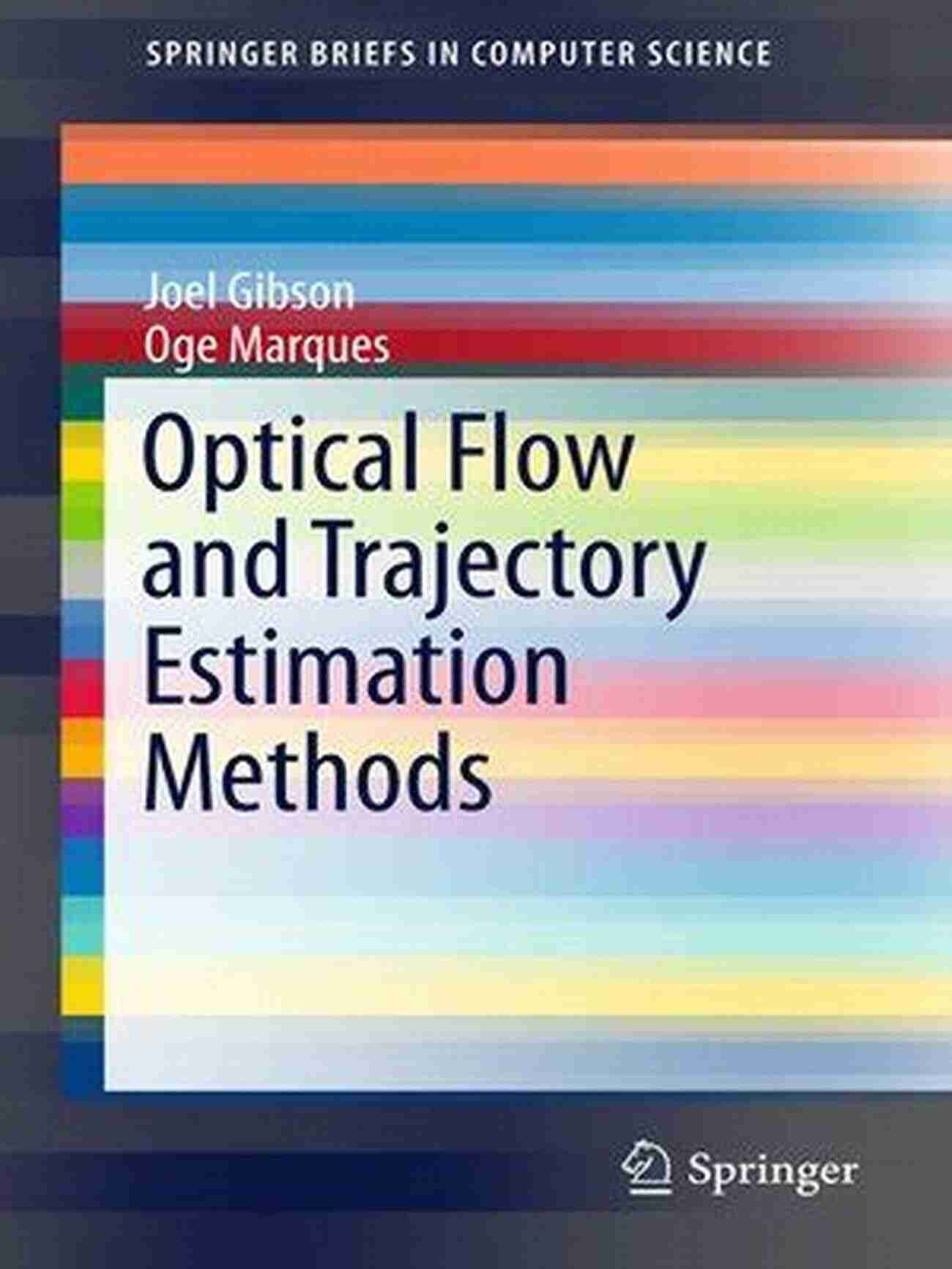
The field of computer vision has witnessed remarkable advancements over the years, and one of the most crucial techniques that has contributed to this progress is optical flow and trajectory estimation methods. In this digital age, where visual data is abundant and essential in various industries, these methods play a pivotal role in tasks such as object tracking, video analysis, autonomous vehicles, and augmented reality.
What is Optical Flow?
Optical flow refers to the pattern of apparent motion of pixels between consecutive frames in a sequence of images or videos. By estimating the optical flow, we can understand the motion dynamics of objects present in the scene. It involves capturing the displacement vectors of pixels from one frame to the next, allowing us to track objects, analyze their movements, and extract valuable information from the visual data.
Importance in Computer Vision
Optical flow estimation acts as a fundamental step in many computer vision applications. By gaining knowledge about the motion patterns of objects, we can enhance the understanding and analysis of visual data. Some key areas where optical flow and trajectory estimation methods are extensively utilized include:
5 out of 5
Language | : | English |
File size | : | 1453 KB |
Text-to-Speech | : | Enabled |
Enhanced typesetting | : | Enabled |
Print length | : | 80 pages |
Screen Reader | : | Supported |
Paperback | : | 114 pages |
Reading age | : | 14 years and up |
Item Weight | : | 12 ounces |
Dimensions | : | 5.59 x 0.67 x 8.19 inches |
- Object Tracking: Optical flow algorithms assist in tracking objects by continuously estimating the motion vectors of pixels within the region of interest. This enables accurate and robust tracking, even in scenarios with occlusions or cluttered backgrounds.
- Video Analysis: By estimating optical flow, we can derive essential information such as object speed, direction, and motion segmentation. This knowledge helps in tasks like action recognition, video summarization, and anomaly detection.
- Autonomous Vehicles: Optical flow algorithms play a crucial role in self-driving cars by perceiving the motion of surrounding objects, predicting their trajectories, and assisting in decision-making processes.
- Augmented Reality: Optical flow estimation aids in aligning virtual objects with the real-world scene, creating seamless and realistic augmented reality experiences.
Popular Optical Flow and Trajectory Estimation Methods
Over the years, several methods have been developed to estimate optical flow and trajectories accurately. Let's explore some of the popular techniques:
Lucas-Kanade Method
The Lucas-Kanade method is a classic optical flow estimation algorithm that assumes a constant intensity pattern within a small neighborhood of pixels. It relies on the brightness constancy assumption and solves for optical flow by minimizing the squared difference between the observed and predicted image gradients.
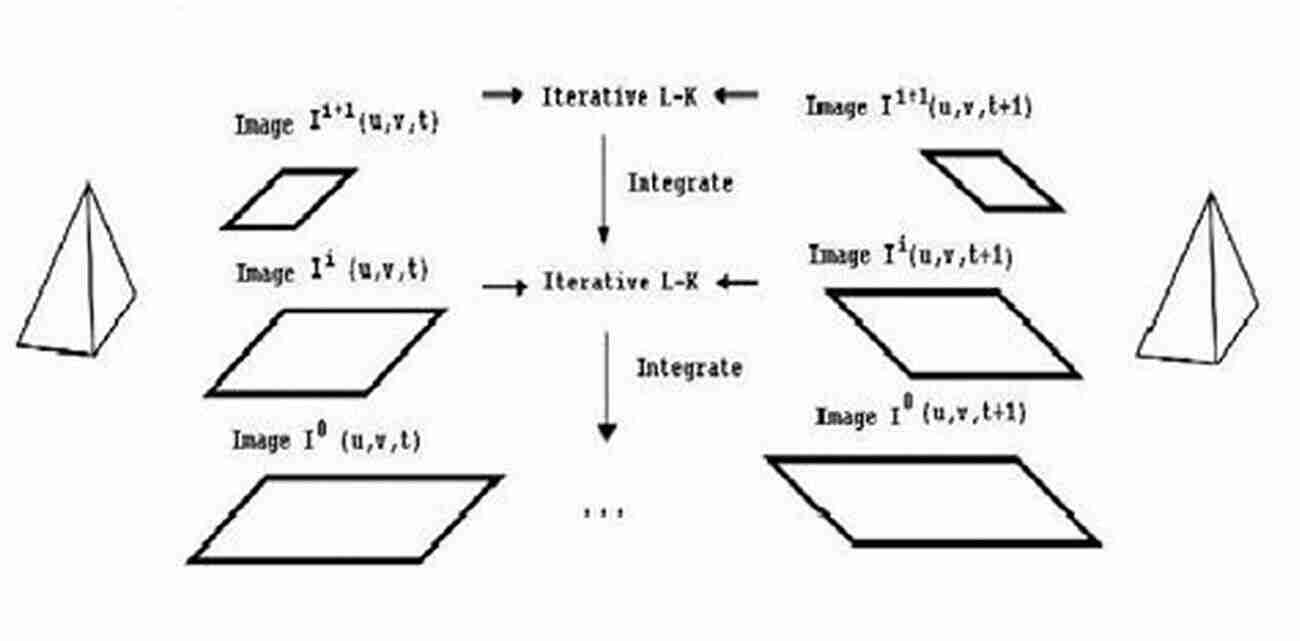
Farneback Method
The Farneback method utilizes polynomial expansion to estimate dense optical flow. It represents the flow as a 2D polynomial function and employs a hierarchical approach to capture motion at different scales.
Deep Learning-based Approaches
With the advent of deep learning, optical flow estimation methods have witnessed significant improvements. Deep neural networks can learn complex motion patterns from large-scale datasets, enabling accurate and robust flow estimation even in challenging scenarios.
Challenges and Future Directions
While optical flow and trajectory estimation methods have revolutionized computer vision, there are still challenges to overcome. Dealing with occlusions, handling large displacements, and achieving real-time performance are areas that researchers continue to address.
Future directions in this domain include the incorporation of semantic information to enhance accuracy, utilizing recurrent neural networks for temporal modeling, and exploring hybrid methods that combine traditional techniques with deep learning approaches.
Optical flow and trajectory estimation methods have paved the way for groundbreaking applications in computer vision. They enable us to decipher complex motion patterns, track objects accurately, and extract valuable insights from visual data. As researchers continue to innovate and refine these methods, further advancements in computer vision will undoubtedly be achieved - unlocking endless possibilities for various industries.
5 out of 5
Language | : | English |
File size | : | 1453 KB |
Text-to-Speech | : | Enabled |
Enhanced typesetting | : | Enabled |
Print length | : | 80 pages |
Screen Reader | : | Supported |
Paperback | : | 114 pages |
Reading age | : | 14 years and up |
Item Weight | : | 12 ounces |
Dimensions | : | 5.59 x 0.67 x 8.19 inches |
This brief focuses on two main problems in the domain of optical flow and trajectory estimation: (i) The problem of finding convex optimization methods to apply sparsity to optical flow; and (ii) The problem of how to extend sparsity to improve trajectories in a computationally tractable way.
Beginning with a review of optical flow fundamentals, it discusses the commonly used flow estimation strategies and the advantages or shortcomings of each. The brief also introduces the concepts associated with sparsity including dictionaries and low rank matrices. Next, it provides context for optical flow and trajectory methods including algorithms, data sets, and performance measurement. The second half of the brief covers sparse regularization of total variation optical flow and robust low rank trajectories. The authors describe a new approach that uses partially-overlapping patches to accelerate the calculation and is implemented in a coarse-to-fine strategy. Experimental results show that combining total variation and a sparse constraint from a learned dictionary is more effective than employing total variation alone.
The brief is targeted at researchers and practitioners in the fields of engineering and computer science. It caters particularly to new researchers looking for cutting edge topics in optical flow as well as veterans of optical flow wishing to learn of the latest advances in multi-frame methods.
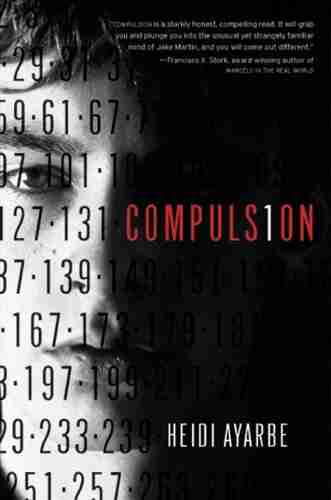

Compulsion Heidi Ayarbe - A Gripping Tale of Addiction...
Compulsion Heidi Ayarbe...
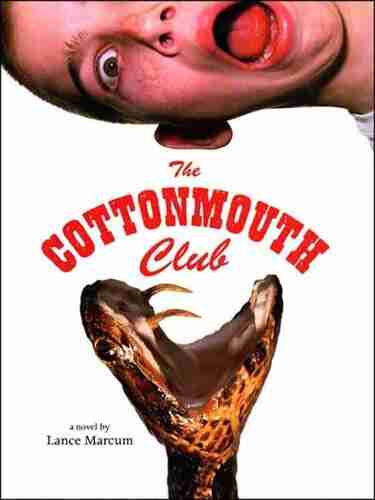

The Cottonmouth Club Novel - Uncovering the Secrets of a...
Welcome to the dark and twisted world of...
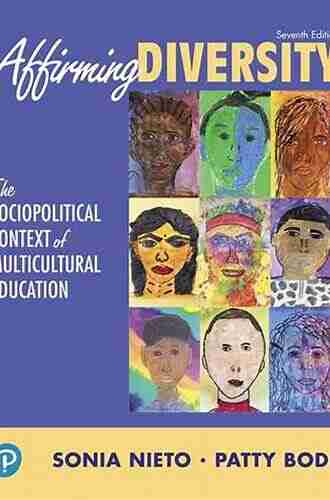

The Sociopolitical Context Of Multicultural Education...
Living in a diverse and interconnected world,...
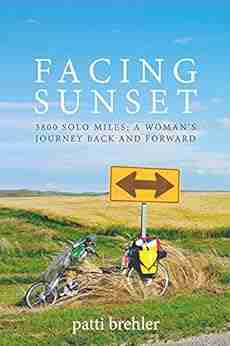

The Epic Journey of a Woman: 3800 Solo Miles Back and...
Embarking on a solo journey is a...
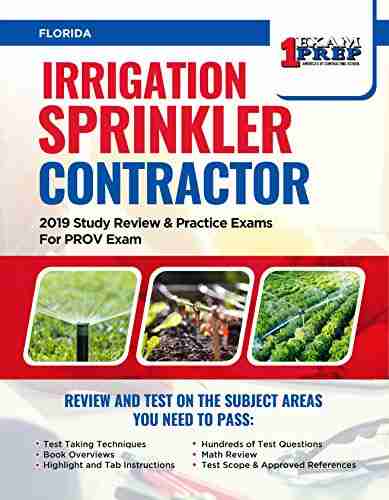

Florida Irrigation Sprinkler Contractor: Revolutionizing...
Florida, known for its beautiful...
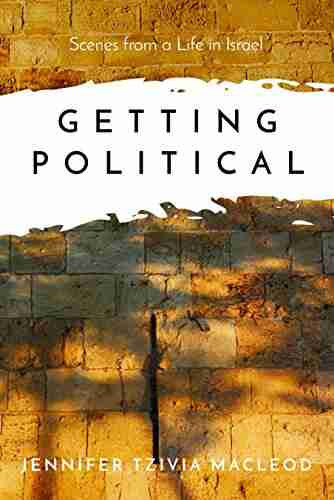

Unveiling the Political Tapestry: Life in Israel
Israel, a vibrant country located in the...
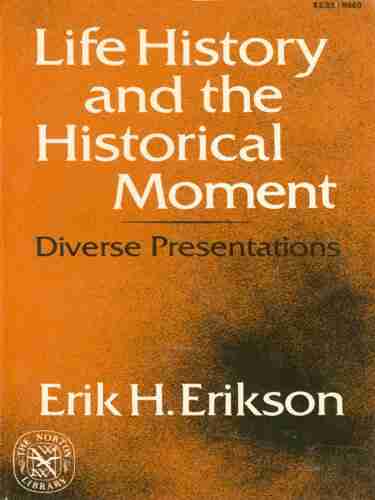

Life History And The Historical Moment Diverse...
Do you ever find yourself...
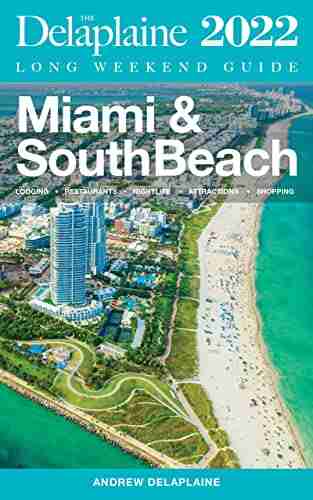

Miami South Beach The Delaplaine 2022 Long Weekend Guide
Welcome to the ultimate guide for...
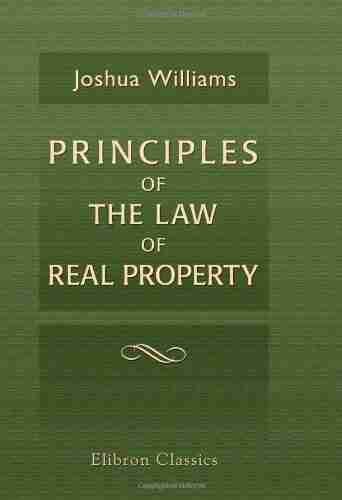

An In-depth Look into the Principles of the Law of Real...
The principles of the...
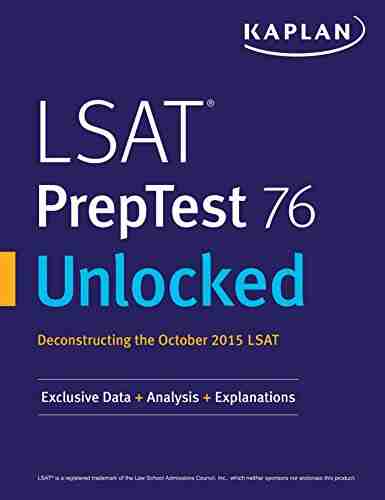

Exclusive Data Analysis Explanations For The October 2015...
Are you preparing for the Law School...
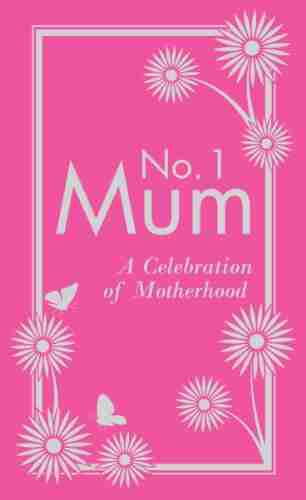

The Secret to Enjoying Motherhood: No Mum Celebration of...
Being a mother is a truly remarkable...
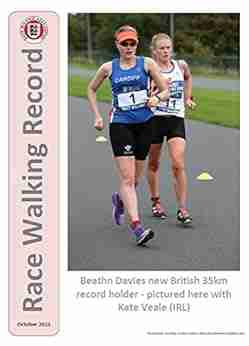

Race Walking Record 913 October 2021
Are you ready for an...
Light bulbAdvertise smarter! Our strategic ad space ensures maximum exposure. Reserve your spot today!
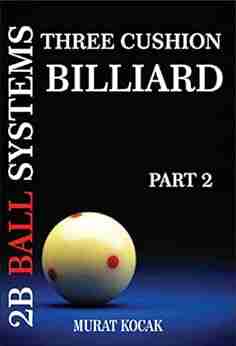

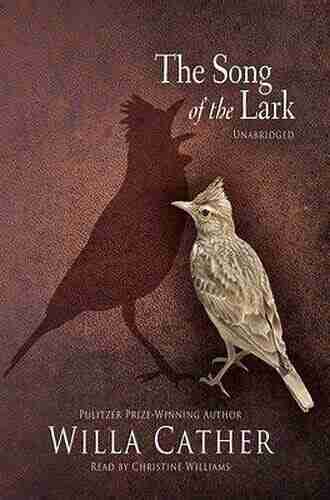

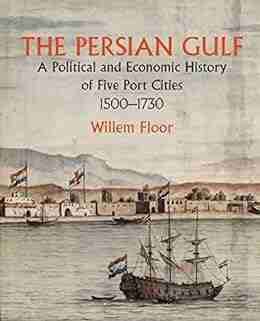

- Ted SimmonsFollow ·17k
- Gerald BellFollow ·11.4k
- Ruben CoxFollow ·19.6k
- Damon HayesFollow ·6.9k
- Richard SimmonsFollow ·15.2k
- Pablo NerudaFollow ·10.2k
- Darnell MitchellFollow ·3k
- J.D. SalingerFollow ·19k