Book Downloads Hub Reads Ebooks Online eBook Librarys Digital Books Store Download Book Pdfs Bookworm Downloads Free Books Downloads Epub Book Collection Pdf Book Vault Read and Download Books Open Source Book Library Best Book Downloads Alan Philips Stephen Woessner Janet Young Raymond E Feist Linda Hutsell Manning Shawn James Jeffery Barrera Ronald D Quinn
Do you want to contribute by writing guest posts on this blog?
Please contact us and send us a resume of previous articles that you have written.
Exploring the Future of Fiber Optic Communication Systems Through Machine Learning

In this era of rapid technological advancements, the demand for faster and more reliable communication systems has never been higher. Enter machine learning, a field of artificial intelligence that has the potential to revolutionize the way we design and optimize fiber optic communication systems for the future.
The Role of Fiber Optic Communication Systems
Fiber optic communication systems form the backbone of modern telecommunications networks, enabling the transmission of vast amounts of data over long distances at incredible speeds. Unlike traditional copper cables, fiber optics use thin strands of glass or plastic to transmit light signals, which can carry data much faster and more efficiently.
These systems are widely used in various sectors, including telecommunication companies, internet service providers, and data centers, as they offer high bandwidth, low signal degradation, and immunity to electromagnetic interference.
4.4 out of 5
Language | : | English |
File size | : | 69817 KB |
Text-to-Speech | : | Enabled |
Enhanced typesetting | : | Enabled |
Print length | : | 176 pages |
Screen Reader | : | Supported |
The Challenges faced by Fiber Optic Communication Systems
While fiber optic technology has revolutionized the way we communicate, it is not without its challenges. As data demands continue to grow exponentially, the optimization and maintenance of these systems become increasingly complex.
One of the major challenges is accurately predicting and diagnosing potential faults or failures in the fiber optic network. Traditionally, these tasks have been carried out by human operators, which can be time-consuming and error-prone.
Moreover, fiber optic networks are subjected to various environmental conditions and external factors, such as temperature, humidity, and physical disturbances, which can affect their performance. Detecting and mitigating these issues in real-time is crucial to ensure uninterrupted communication.
How Machine Learning Empowers Fiber Optic Communication Systems
Machine learning offers a promising solution to overcome the challenges faced by fiber optic communication systems. By leveraging computational models and algorithms, machine learning can analyze large sets of data in real-time, identify patterns, and make accurate predictions.
One of the key applications of machine learning in fiber optics is predictive maintenance. By constantly monitoring the performance of the network and analyzing historical data, machine learning models can detect anomalies and predict potential failures before they occur. This enables proactive maintenance, reducing downtime and improving overall system reliability.
Additionally, machine learning can optimize system performance by dynamically adjusting various parameters based on real-time network conditions. For example, machine learning algorithms can optimize the transmission power, wavelength, and data rate to maximize bandwidth utilization and minimize signal degradation.
The Future of Fiber Optic Communication Systems with Machine Learning
The marriage of machine learning and fiber optic communication systems has the potential to revolutionize the way we communicate and exchange information. As machine learning algorithms become more sophisticated and powerful, they can further enhance the performance, efficiency, and resilience of fiber optic networks.
For instance, machine learning algorithms can learn from historical data to predict the future demand for bandwidth and dynamically allocate resources accordingly. They can also adapt to changing network conditions to optimize performance and mitigate issues.
Furthermore, machine learning can facilitate the development of intelligent and autonomous optical networks. These networks can self-adjust, self-heal, and self-optimize, adapting to traffic patterns and dynamically routing data to ensure efficient and reliable communication.
Machine learning holds tremendous potential for the future of fiber optic communication systems. By leveraging the power of artificial intelligence, we can design, optimize, and operate these systems in ways that were previously unimaginable.
As the demand for high-speed and reliable communication continues to grow, machine learning will play a pivotal role in meeting these needs and driving innovation in the field. It is an exciting time to be a part of this journey as we unlock the true potential of fiber optic communication systems for a connected future.
4.4 out of 5
Language | : | English |
File size | : | 69817 KB |
Text-to-Speech | : | Enabled |
Enhanced typesetting | : | Enabled |
Print length | : | 176 pages |
Screen Reader | : | Supported |
Machine Learning for Future Fiber-Optic Communication Systems provides a comprehensive and in-depth treatment of machine learning concepts and techniques applied to key areas within optical communications and networking, reflecting the state-of-the-art research and industrial practices. The book gives knowledge and insights into the role machine learning-based mechanisms will soon play in the future realization of intelligent optical network infrastructures that can manage and monitor themselves, diagnose and resolve problems, and provide intelligent and efficient services to the end users.
With up-to-date coverage and extensive treatment of various important topics related to machine learning for fiber-optic communication systems, this book is an invaluable reference for photonics researchers and engineers. It is also a very suitable text for graduate students interested in ML-based signal processing and networking.
- Discusses the reasons behind the recent popularity of machine learning (ML) concepts in modern optical communication networks and the why/where/how ML can play a unique role
- Presents fundamental ML techniques like artificial neural networks (ANNs),support vector machines (SVMs),K-means clustering, expectation-maximization (EM) algorithm, principal component analysis (PCA),independent component analysis (ICA),reinforcement learning, and more
- Covers advanced deep learning (DL) methods such as deep neural networks (DNNs),convolutional neural networks (CNNs),recurrent neural networks (RNNs),and generative adversarial networks (GANs)
- Individual chapters focus on ML applications in key areas of optical communications and networking
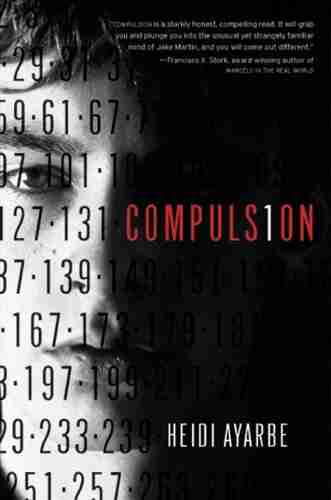

Compulsion Heidi Ayarbe - A Gripping Tale of Addiction...
Compulsion Heidi Ayarbe...
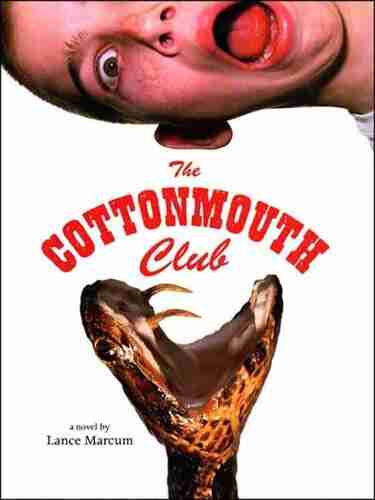

The Cottonmouth Club Novel - Uncovering the Secrets of a...
Welcome to the dark and twisted world of...
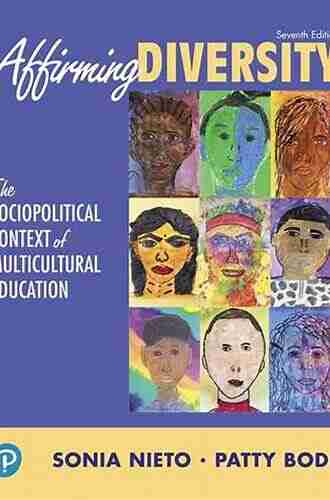

The Sociopolitical Context Of Multicultural Education...
Living in a diverse and interconnected world,...
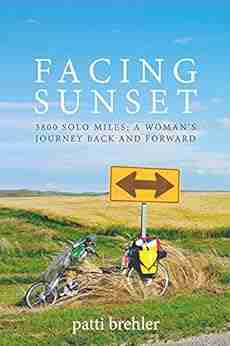

The Epic Journey of a Woman: 3800 Solo Miles Back and...
Embarking on a solo journey is a...
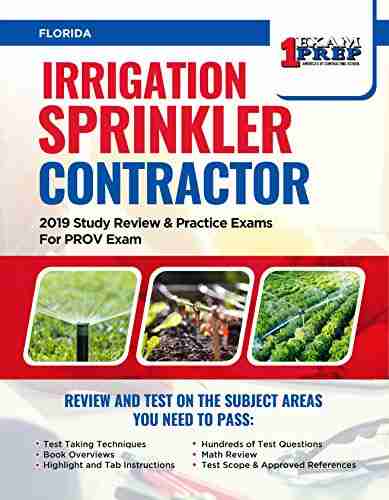

Florida Irrigation Sprinkler Contractor: Revolutionizing...
Florida, known for its beautiful...
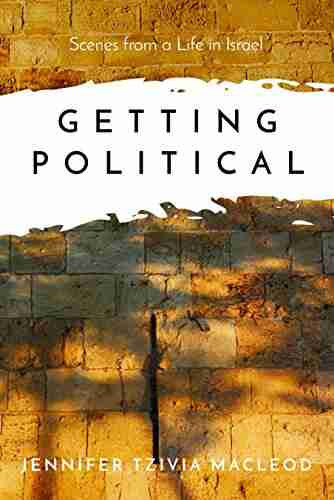

Unveiling the Political Tapestry: Life in Israel
Israel, a vibrant country located in the...
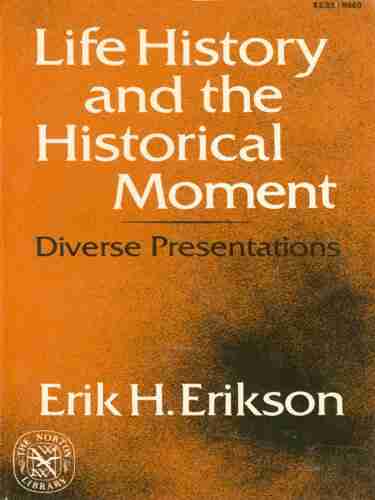

Life History And The Historical Moment Diverse...
Do you ever find yourself...
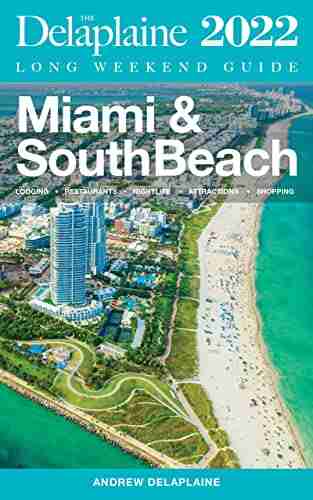

Miami South Beach The Delaplaine 2022 Long Weekend Guide
Welcome to the ultimate guide for...
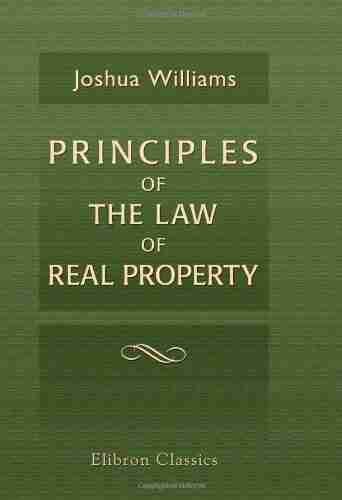

An In-depth Look into the Principles of the Law of Real...
The principles of the...
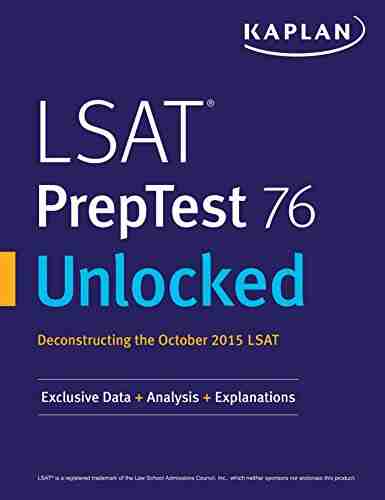

Exclusive Data Analysis Explanations For The October 2015...
Are you preparing for the Law School...
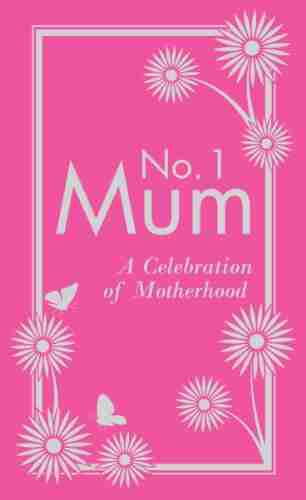

The Secret to Enjoying Motherhood: No Mum Celebration of...
Being a mother is a truly remarkable...
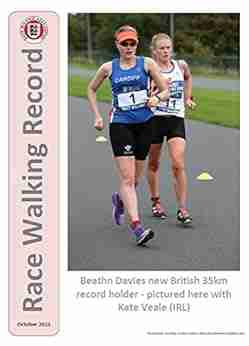

Race Walking Record 913 October 2021
Are you ready for an...
Light bulbAdvertise smarter! Our strategic ad space ensures maximum exposure. Reserve your spot today!
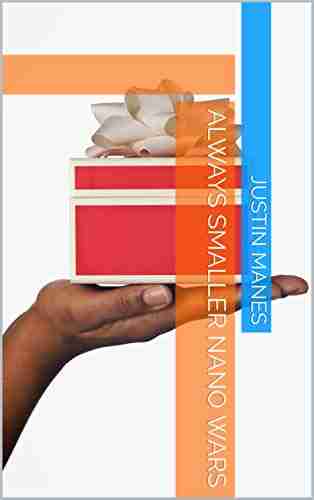

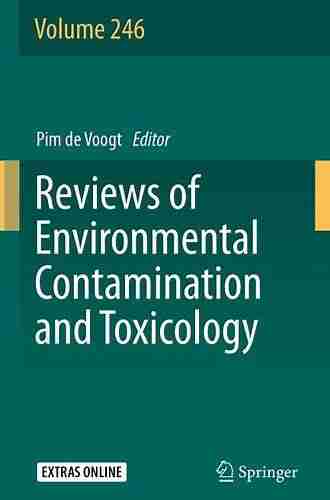

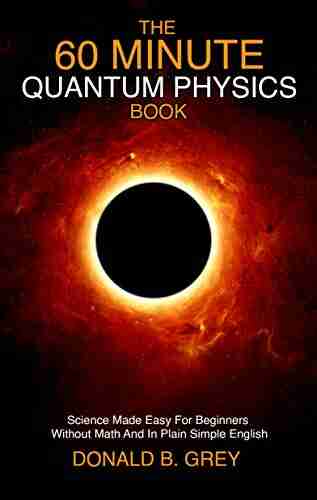

- Martin CoxFollow ·19.4k
- Joseph FosterFollow ·4.7k
- Bradley DixonFollow ·5.2k
- Dwayne MitchellFollow ·5.3k
- Billy PetersonFollow ·17.9k
- Cooper BellFollow ·12.1k
- George R.R. MartinFollow ·6k
- Hugh ReedFollow ·13.1k